Complex Christmas at Lisbon
Yet another christmas at complex networks, this time in Lisbon, a city with rather tastefull character and cods. The stunning venue, Calouste Gulbenkian Foundation is located near the center of Lisbon and is surrounded by an amazing garden, while 5 minutes away there is the beutifull Parque Eduardo VII.
This year I was presenting our online application for scientometrics , but I was not alone. My labmates Changmin Wu and Sammy Khalife presented also their works on matching node embeddings and global capital-ownership networks respectively, and Prof. Vazirgiannis presented a keynote on graph kernels. I attended most of the keynotes and the session around network dynamics and epidemics, so this is a brief overview of the highlights.
Network-based dynamic modeling of bological systems
Dr. Reka Albert presented her group’s work on detecting attractors in biological networks. In that setting each node is a population of molecules and they can have a boolean regulatory state. The future state of a node depends on the current state of its regulators. The main idea is to focus on stable motifes: strongly connected components that represent cyclic sufficient relationships that self maintain themselves and do not change based on the rest of the network. By intervening in the state of these motifs, one can guide the simulation in specific attractor points when the network reaches a steady state. This can be very useful in targeted cell therapy ,because a stable motif will not change even after you change something in the network i.e. inhibition. In other words, more than one interventions may be needed if the network has a stable motif.
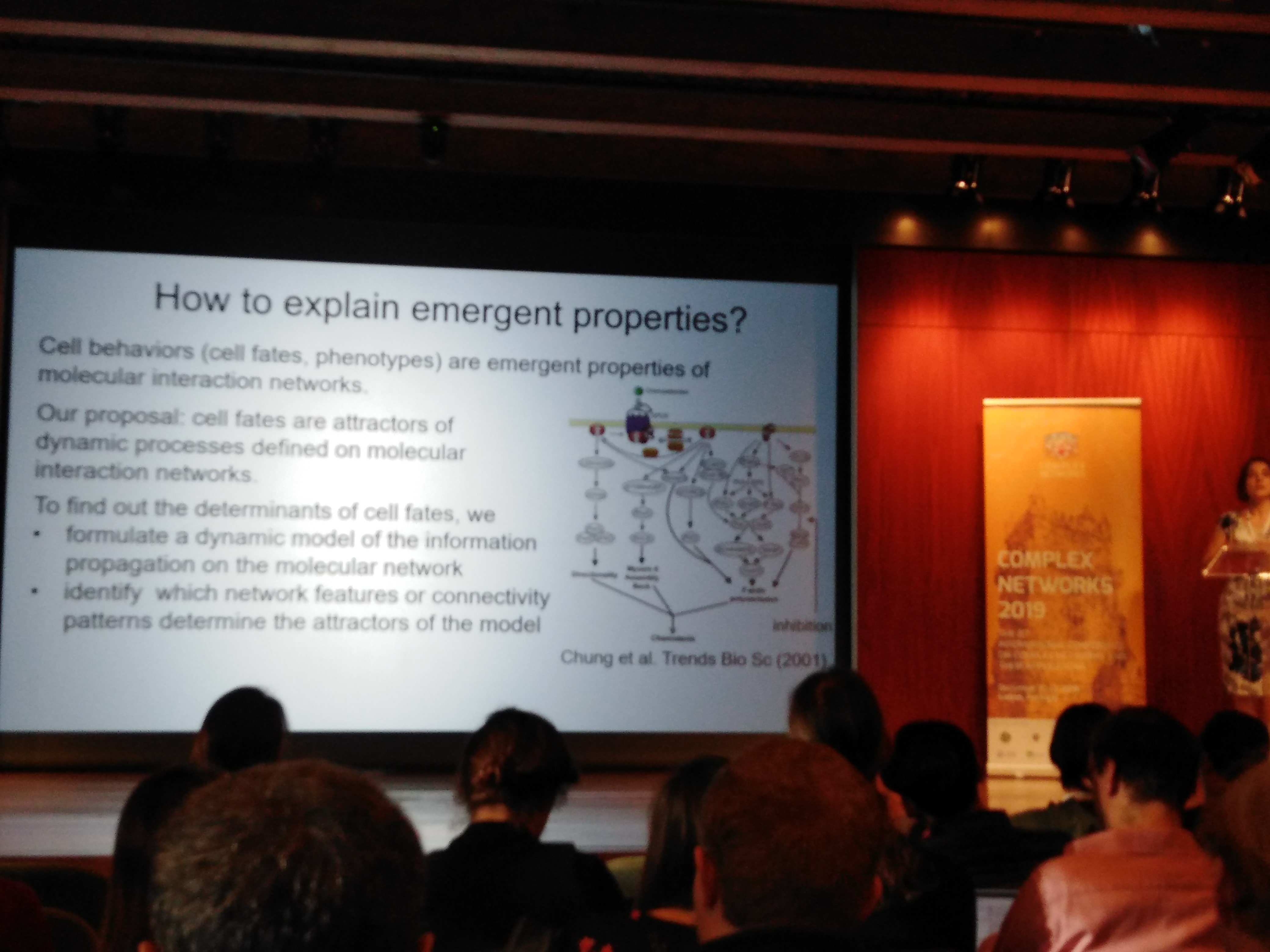
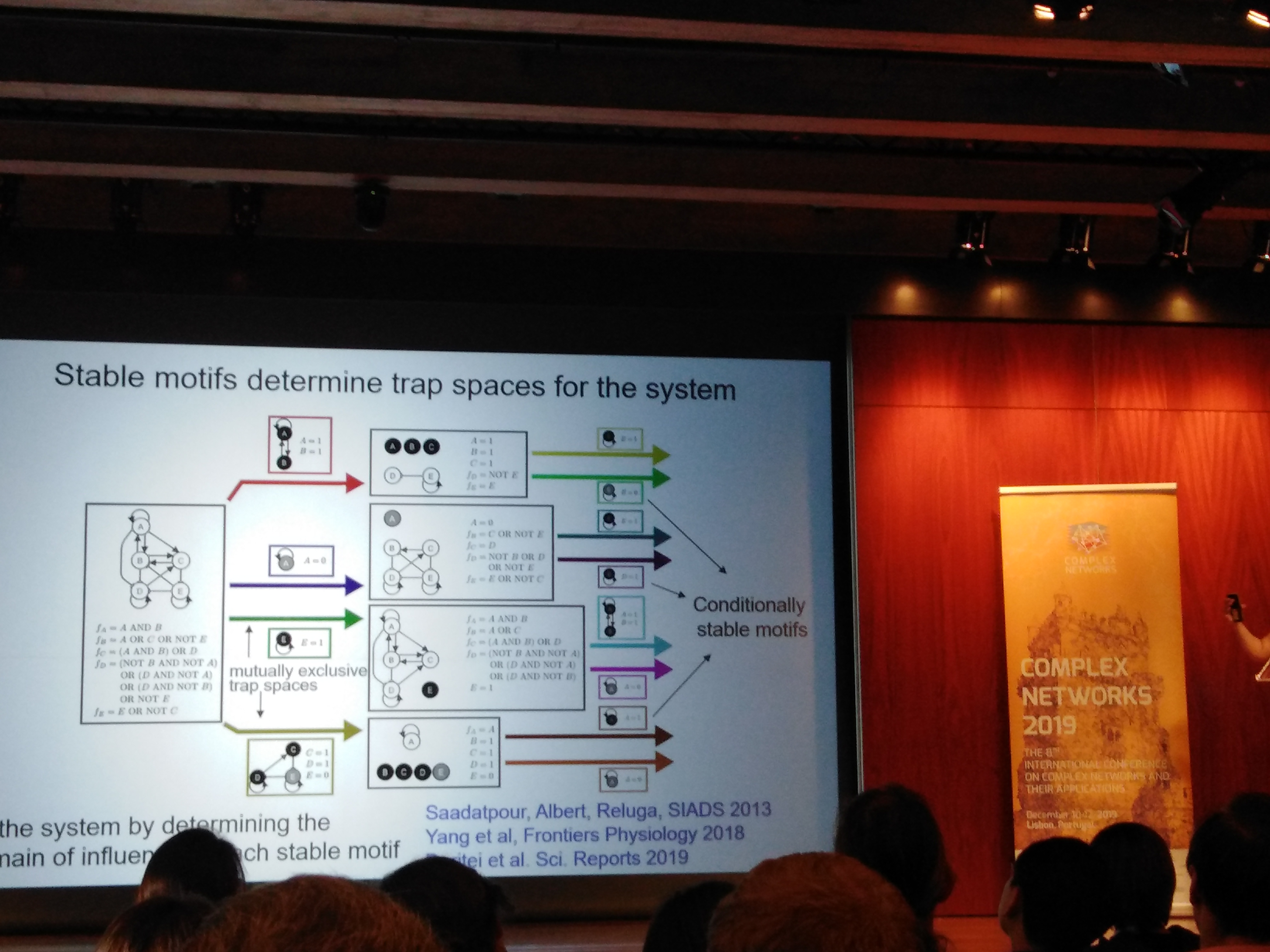
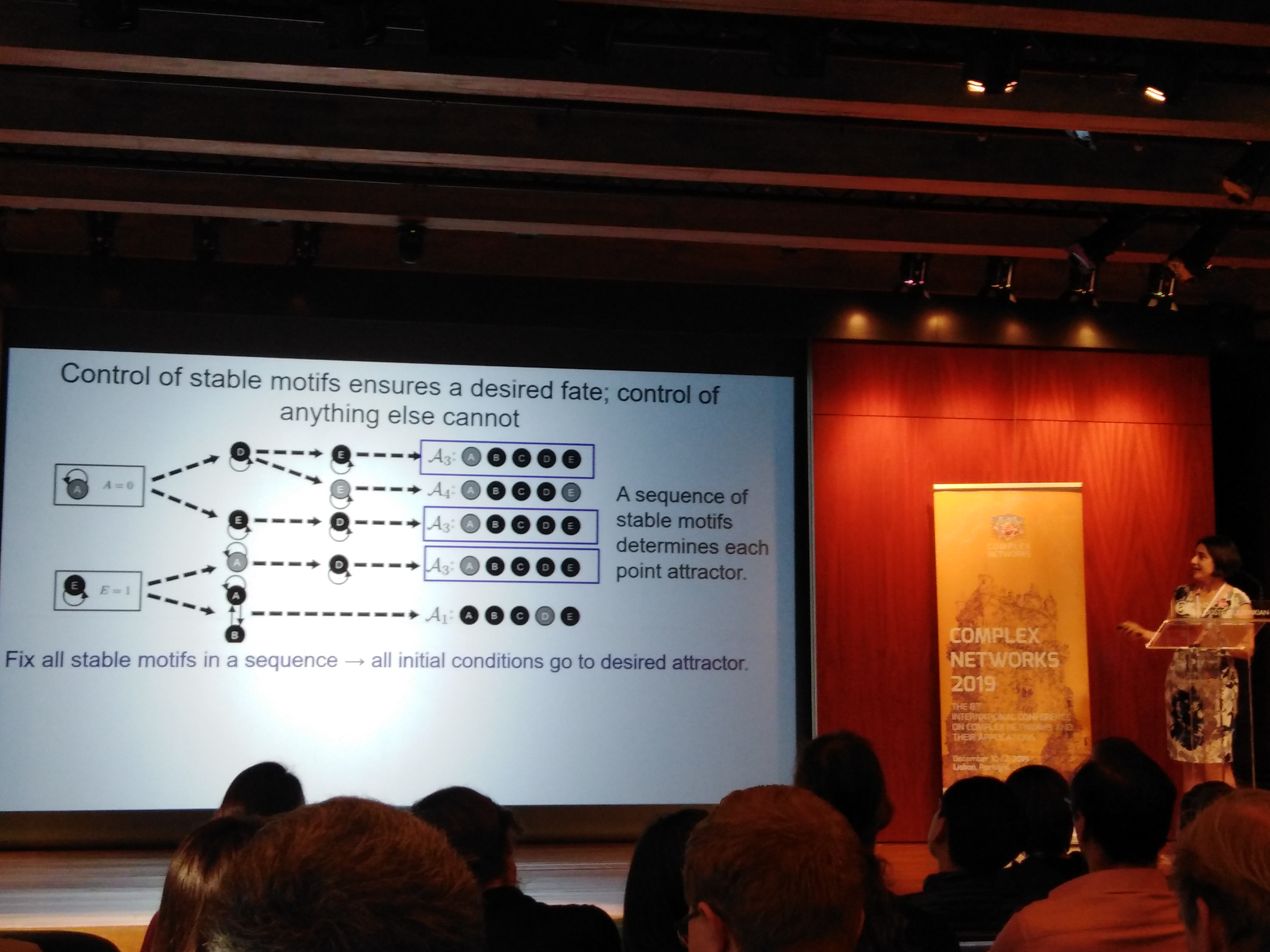
Real-world reflections on online frienshdips
Dr. Lada Adamic presented two distinct exploratory works that showcase patterns of the real world depicted in online friendships.
The first part was an analysis of friendship ties between people in different states of US, which revealed bonds that date back generations ago due to migrations, such as the one from Minessota to California. The same intriguing result applies to country-level, where one could detect heavy connections between Italy and New York, as well as Mexico and California. Overall, it was prevalent how migrants reduce the shortest path of the network.
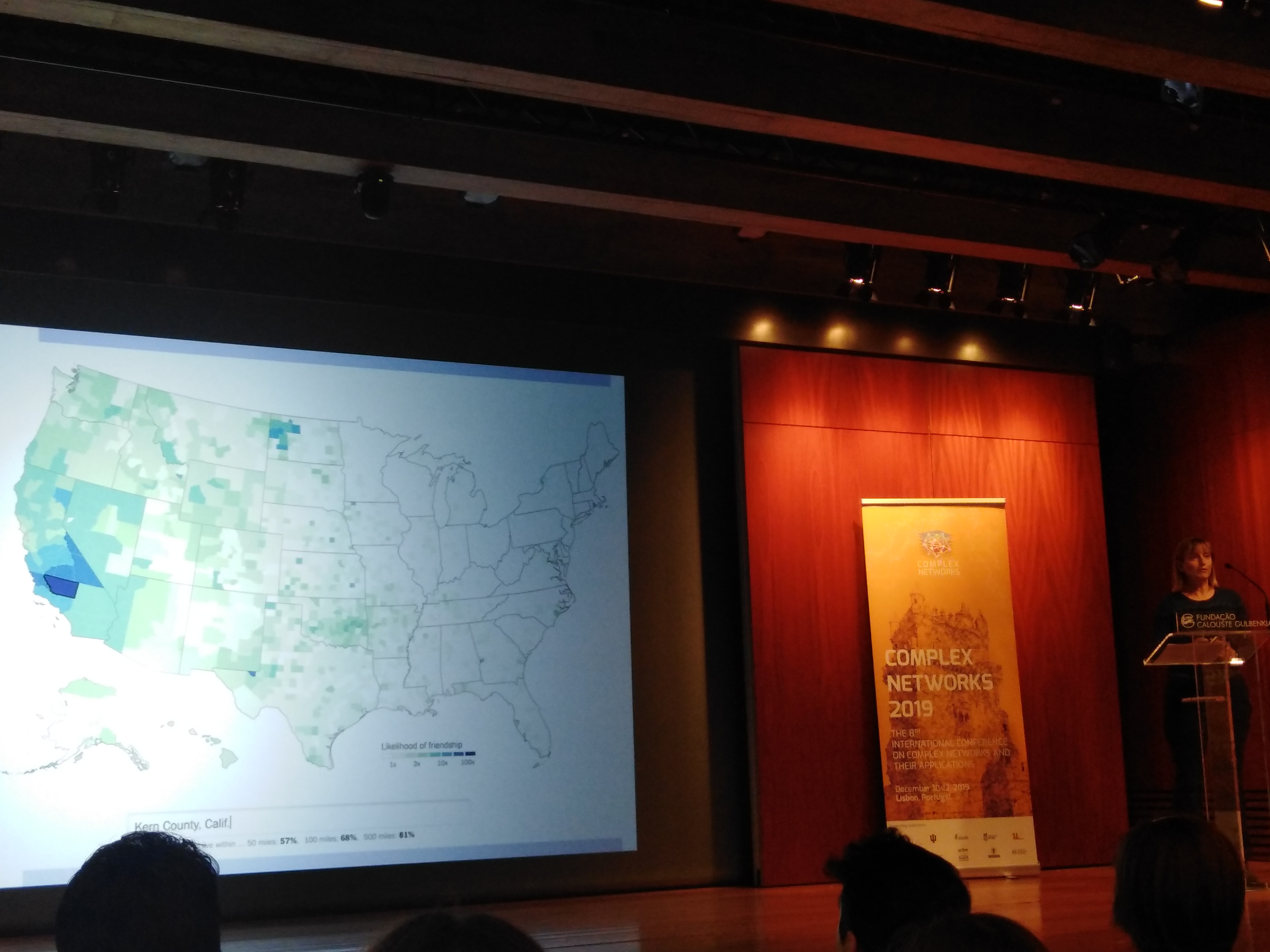
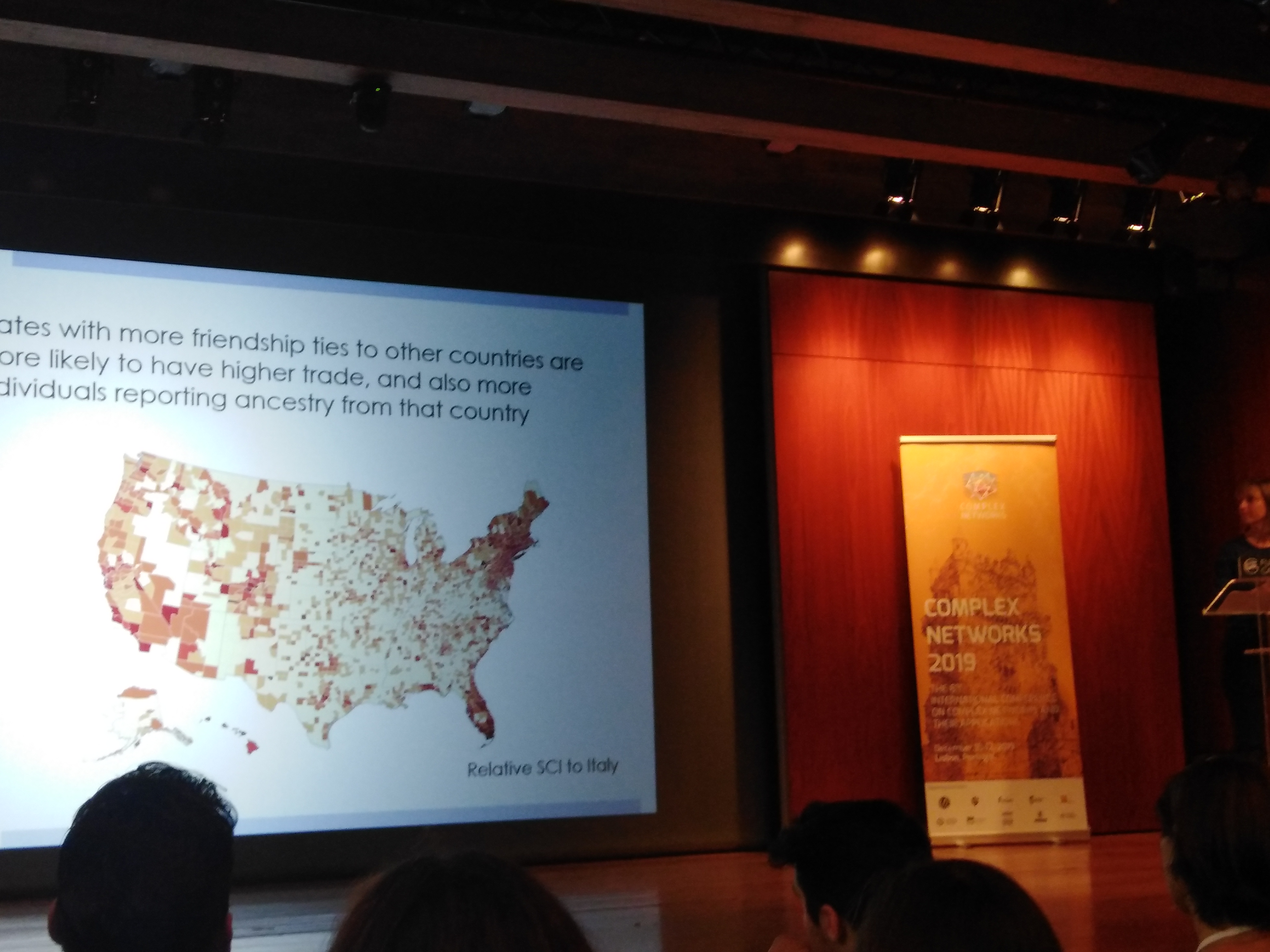
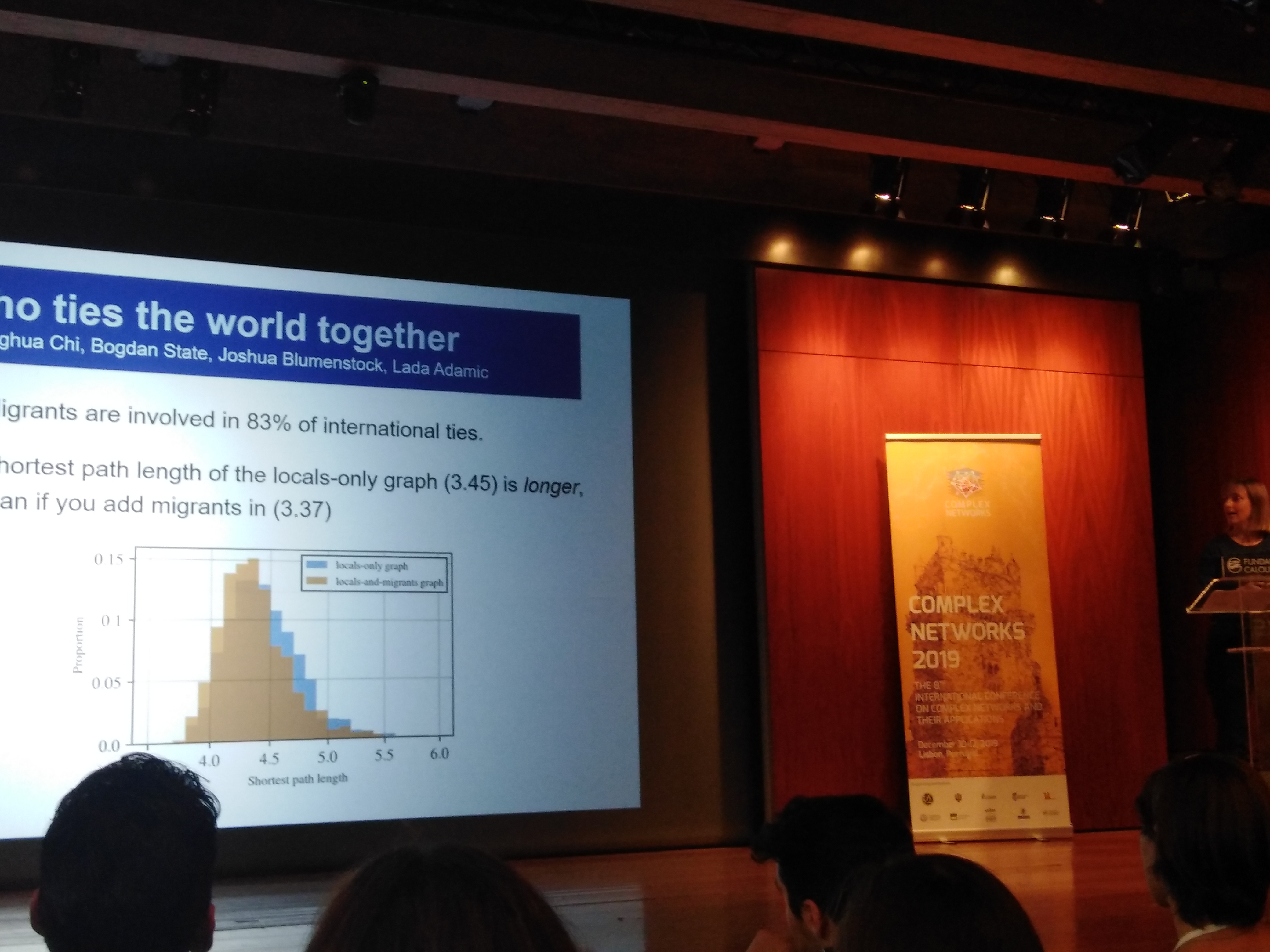
The second part was revolving arround online friendships in college. The exploration answered questions such as when do we make most of our friends in our college life and when is our network stabilizing. Also whether we continue to keep in touch with our college friends. One of the most outstanding results is that the class friendship structure could be a strong predictor of some characteristics of the college.
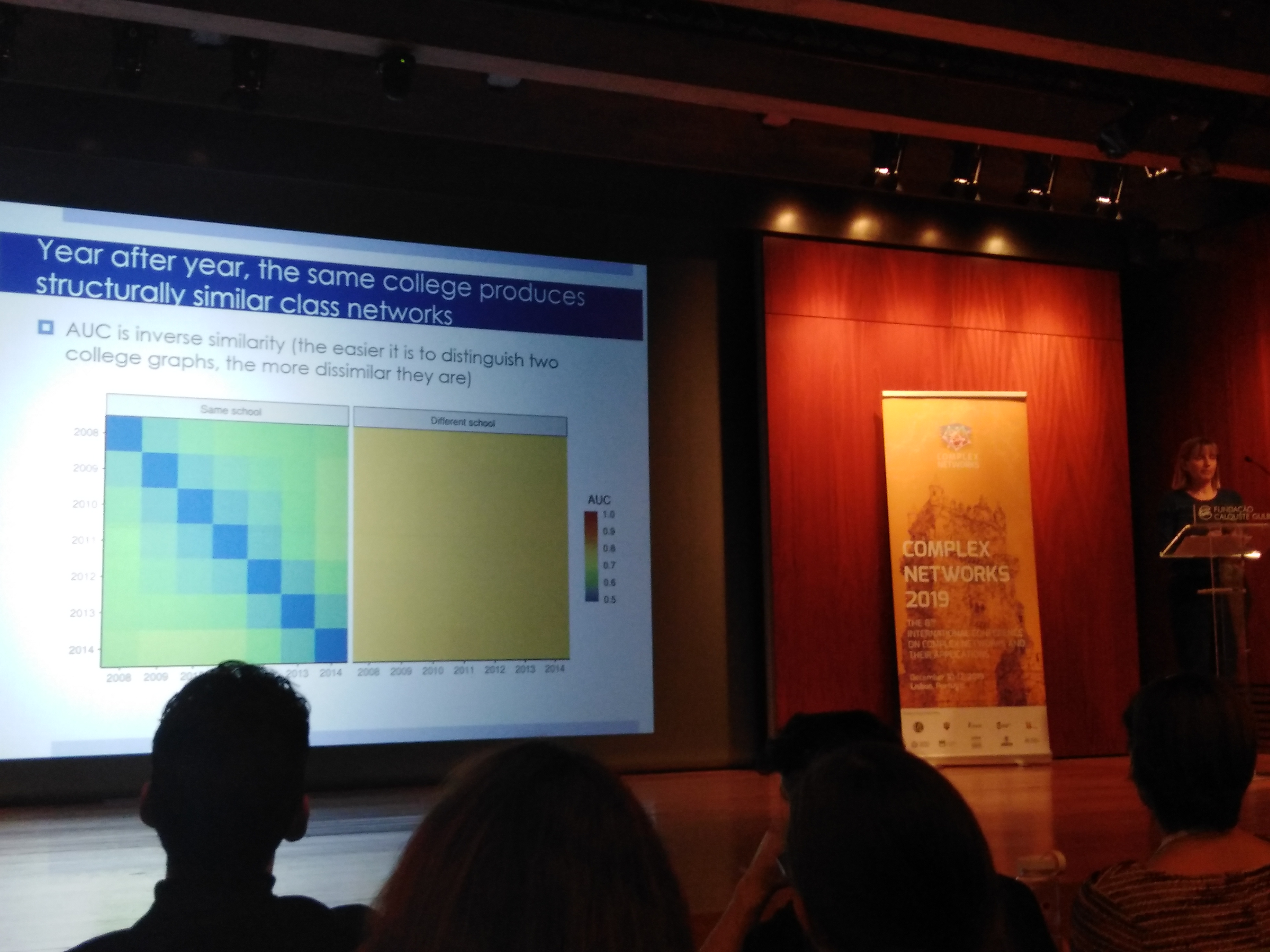
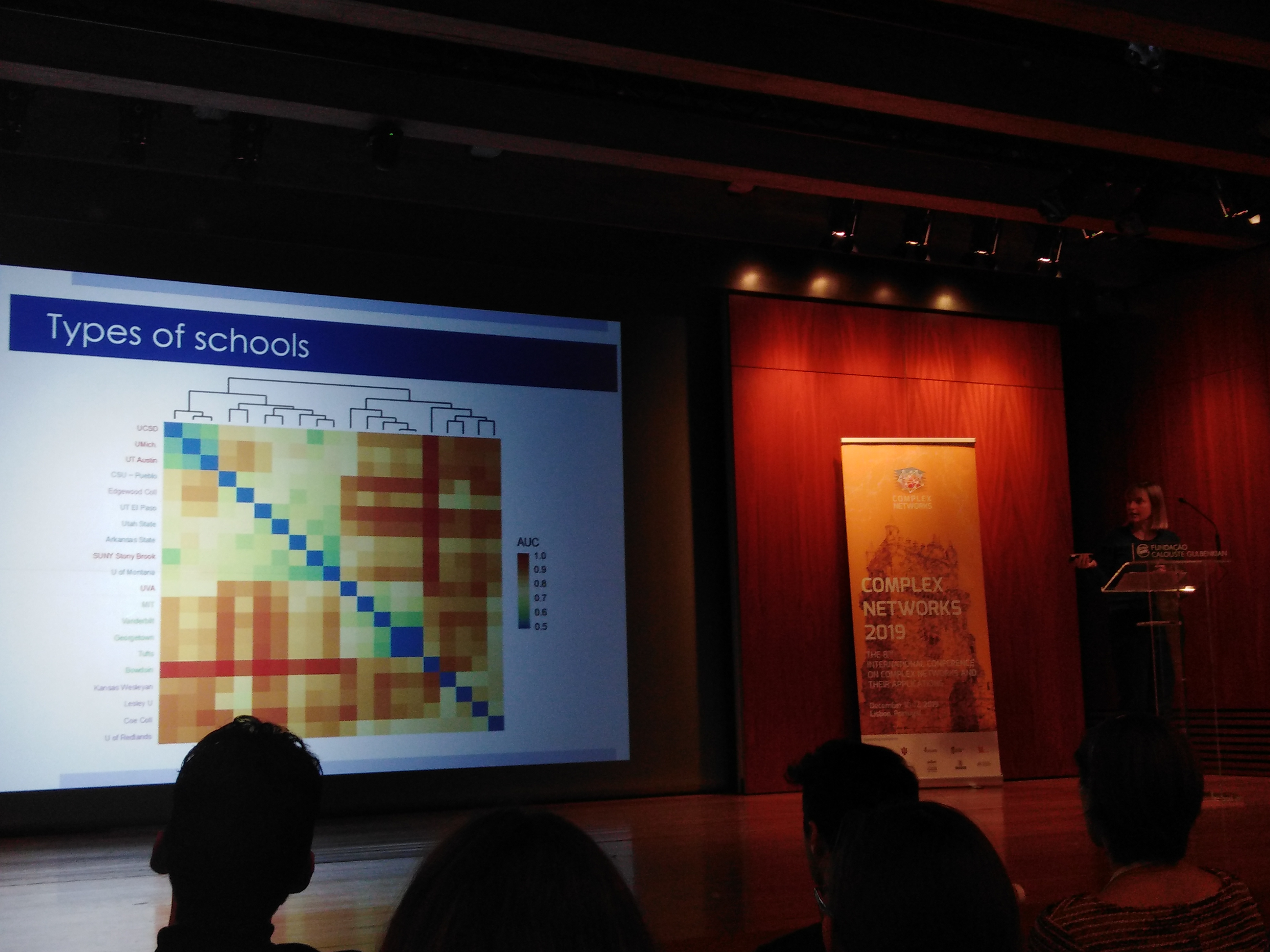
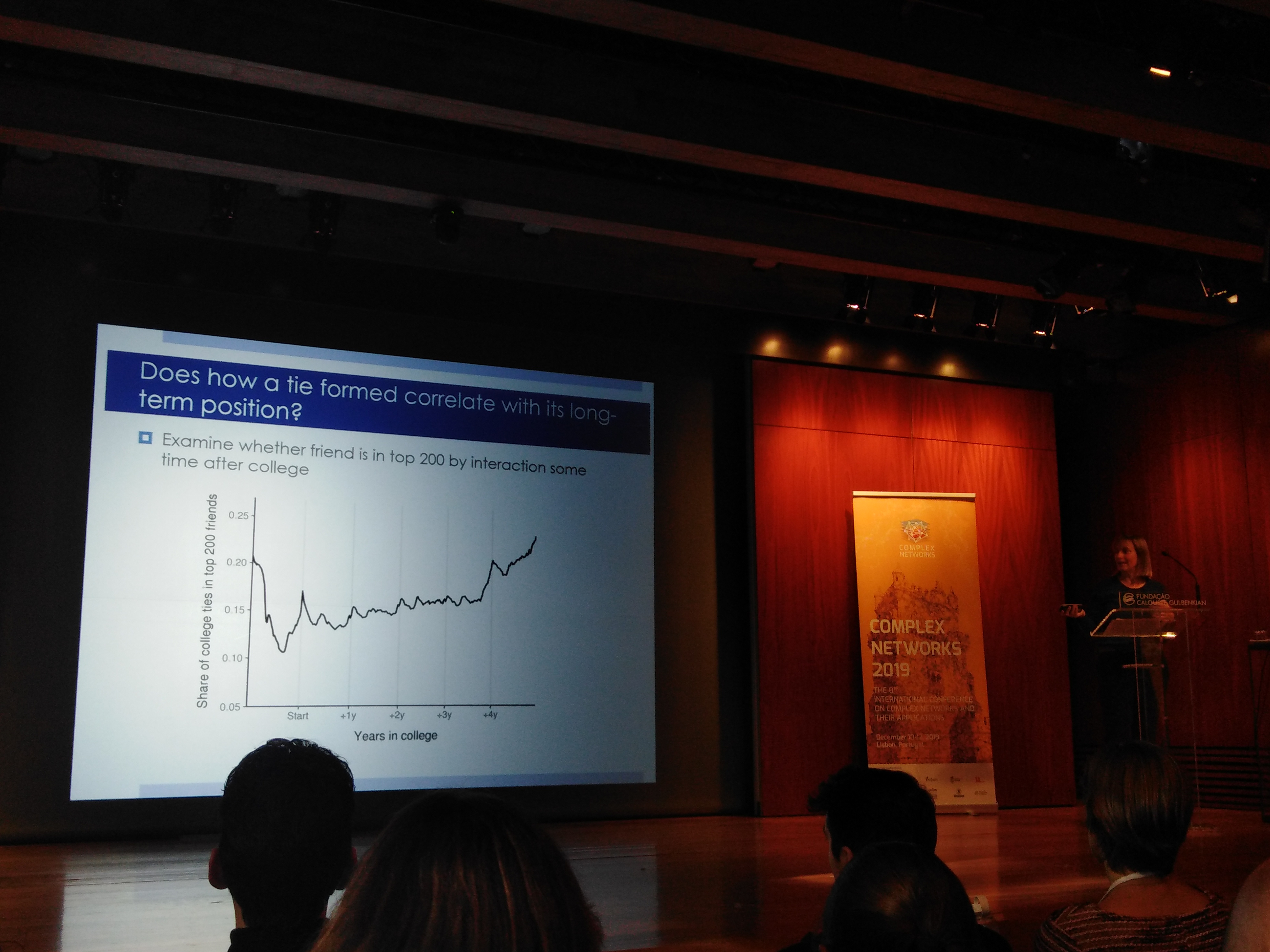
Graph kernels for machine learning tasks
Prof. Vazirgiannis delineated the field of graph kernels, one of the most dominant methods to perform machine learning tasks on graph input. The introduction was an overview of the field and the most easily interpretable kernels, like random walk and shortest path, with small concrete examples. Subsequently, the weisfeiler-lehman test of isomorphism was explained in detail, through a toy example, to provide intuition about the respective kernel. It should be noted that this algorithm has become very prominent lately due to its connection with Graph Neural Networks. Subsequently, other advanced kernels were explained through examples, such as Earth Mover’s Distance and Pyramid Match Graph Kernel, and a benchmarking study using to our team’s new library for graph kernels, GraKel, facilitated a thorough comparison between them. Finally, Prof. Vazirgiannis underlined some key applications of graph kernels,
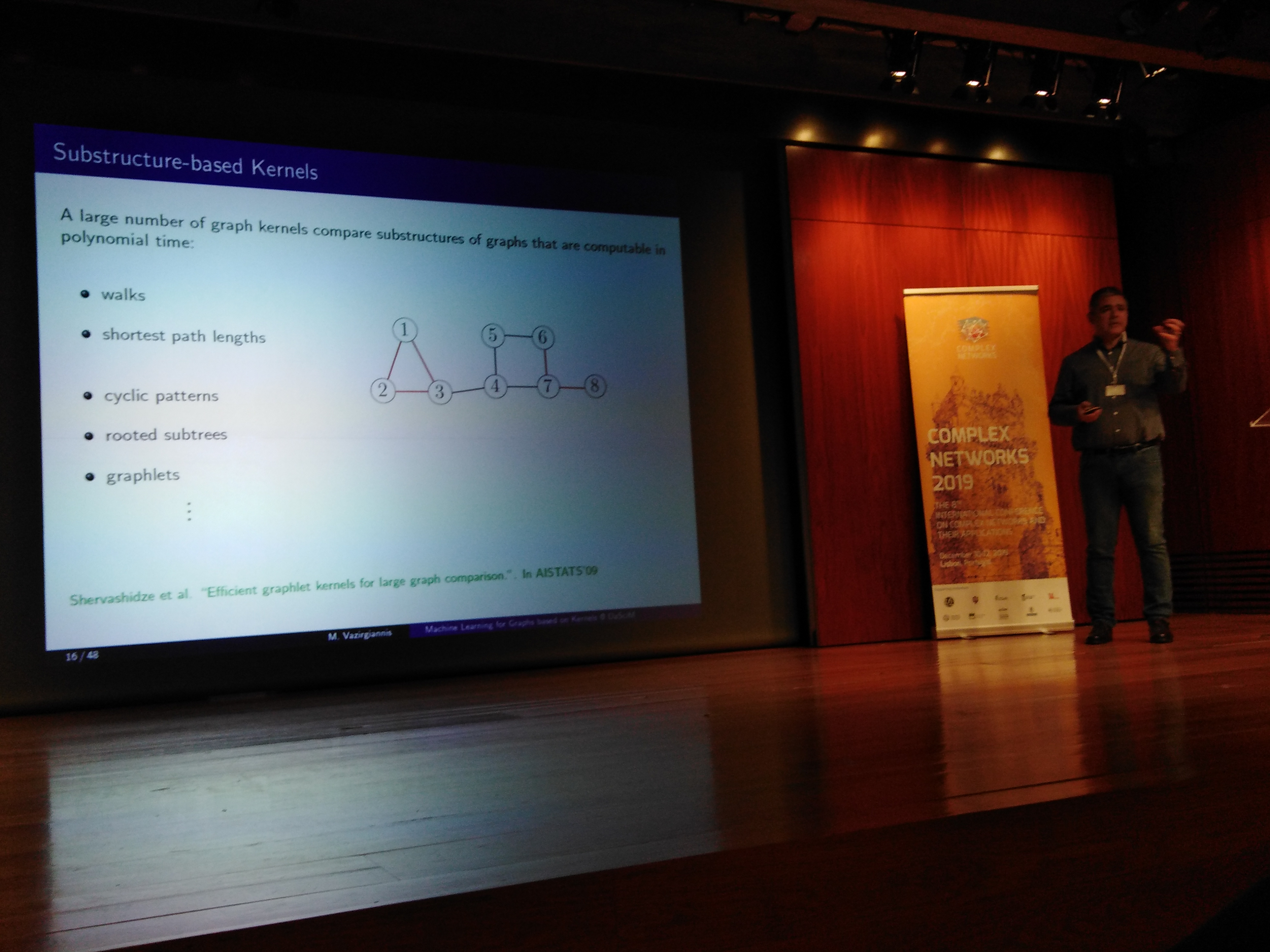
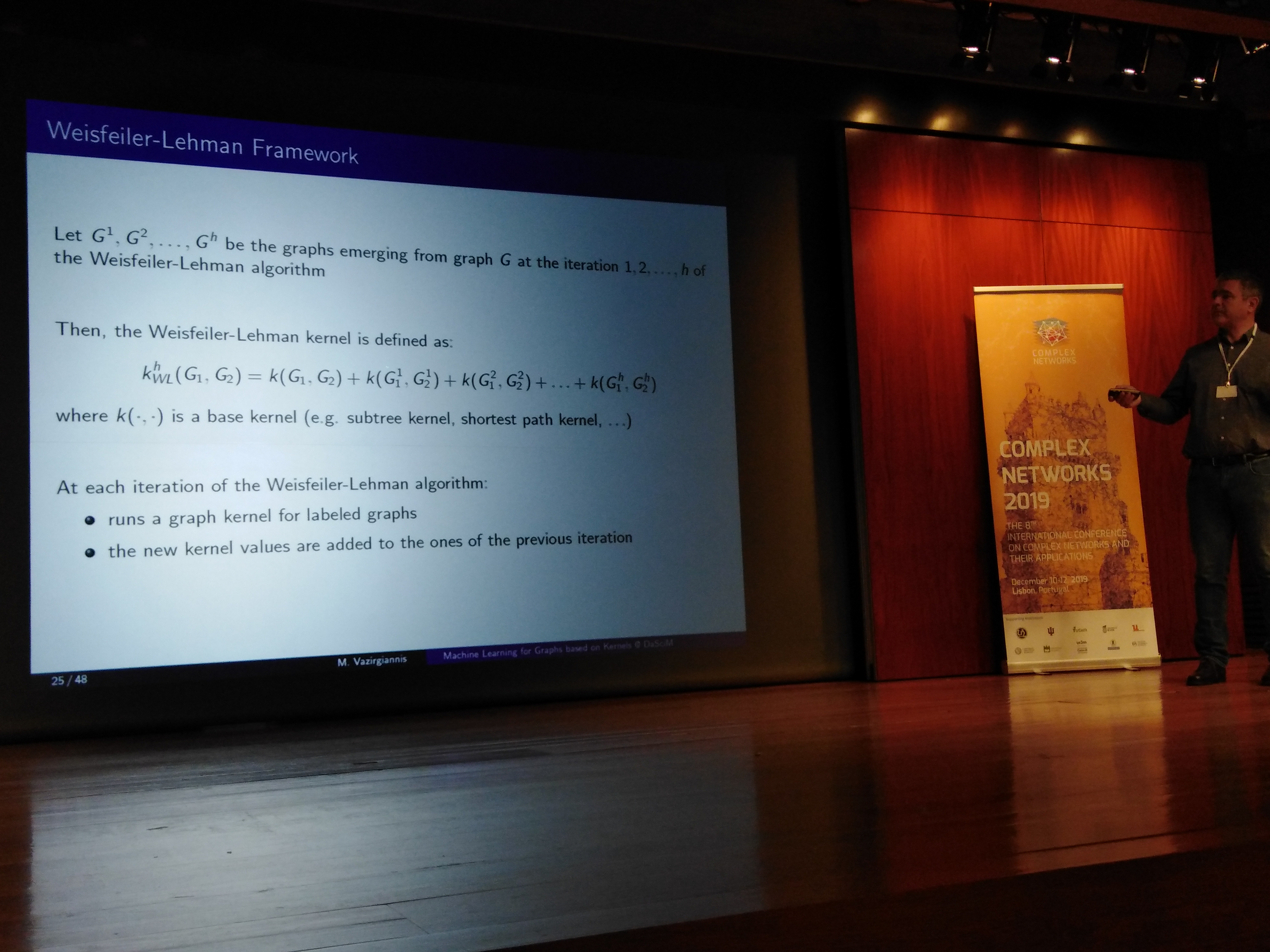
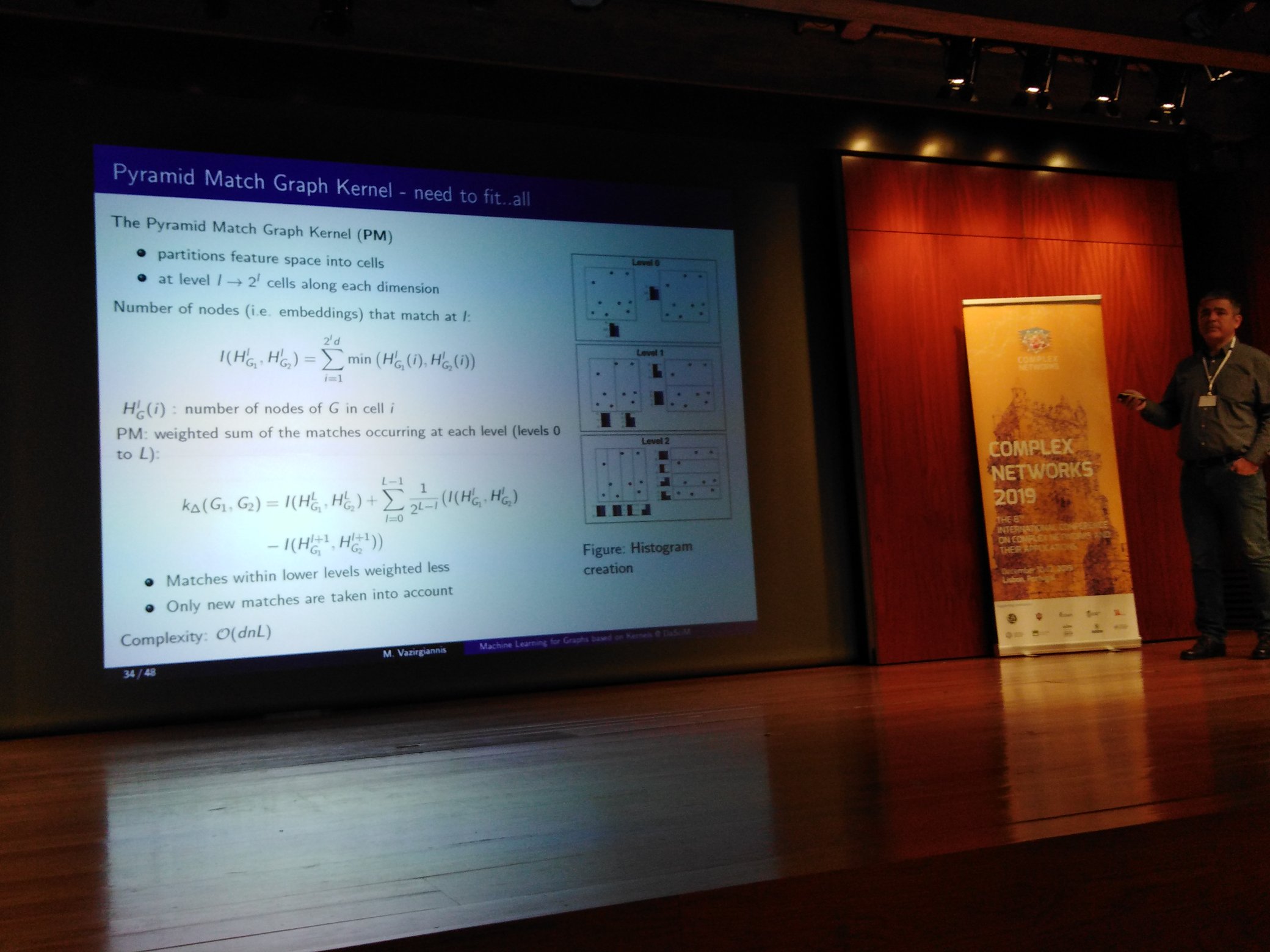
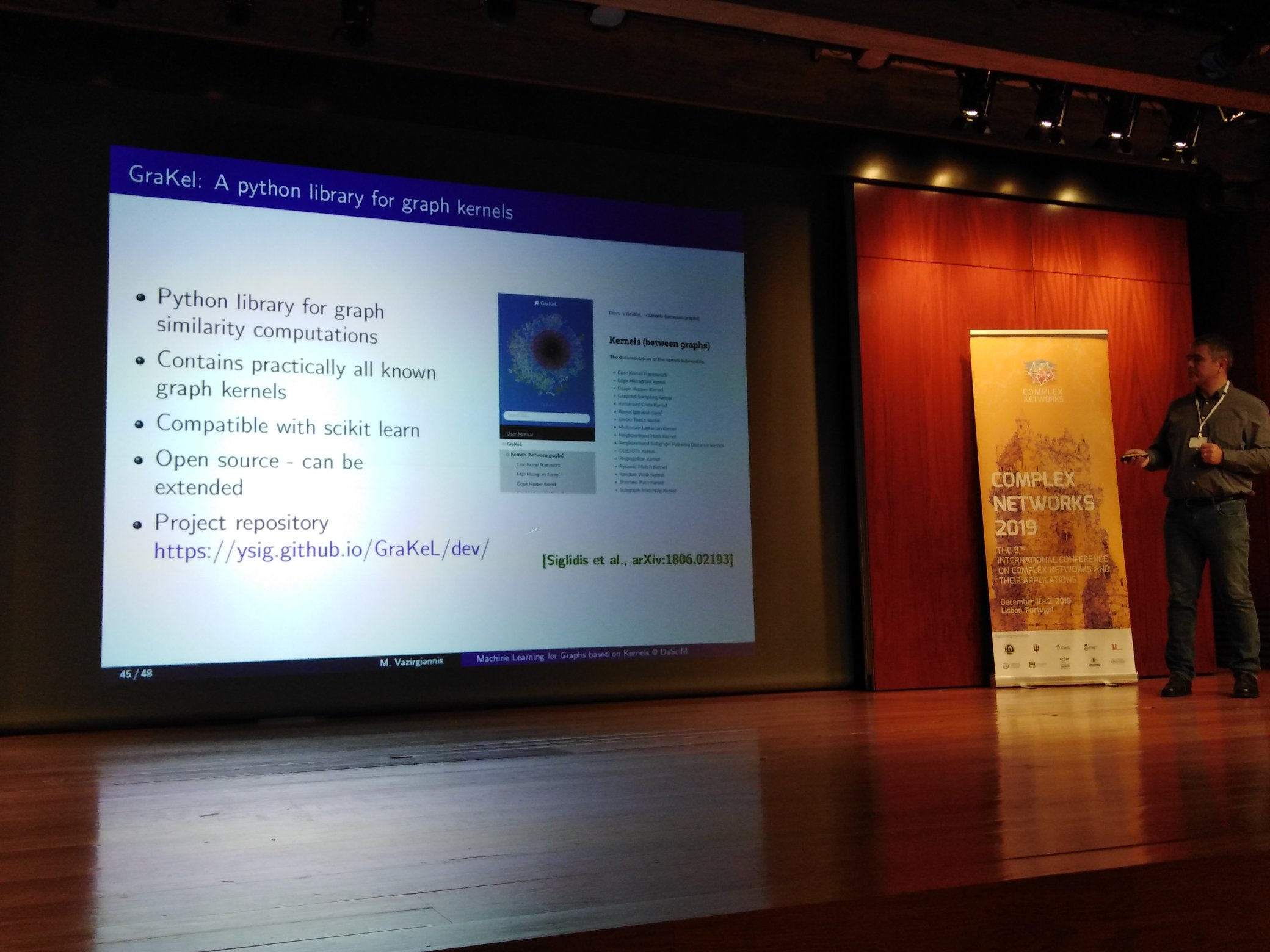
Temporal networks: past, present, future
Prof. Saramaki gave as a full yet interpretable journey to the timeline of temporal networks, based on his recent textbook with Peter Holme. It contained detailed discriptions regarding the different models employed to work with temporal networks (higher order models, event graphs etc.) as well as cases when these approaches are usefull in the real world, one example being detecting causal paths of events in time. Certain observations derived from temporal network analysis were highlighted, such as the well-known burstiness and its effect on spreading. Prof. Saramaki also underlined the methodology of evaluating the effect of structural characteristics in temporal networks by suffling them in time i.e. the temporal reference model. The talk wrapped up by highlighting the strength of this approach in analyzing temporal events in social interactions, and sketched some future directions. Slides available here.
Sampling on Networks: Estimating Eigenvector Centrality on Incomplete Network
Nicolo Ruggeri presented an interested work in approximating eigenvector centrality for networks that are incomplete or too big to fit in a machine. The algorithm has a theoretical guarantee, obtained by bounding the difference between the eigenvector centrality through sampling and the real one. The sampling is done in a greedy manner by adding the node that is a neighbor of the existing nodes and minimizes the aforementioned bounds. Experimental results in simulated and real world networks showcase the method’s superiority.
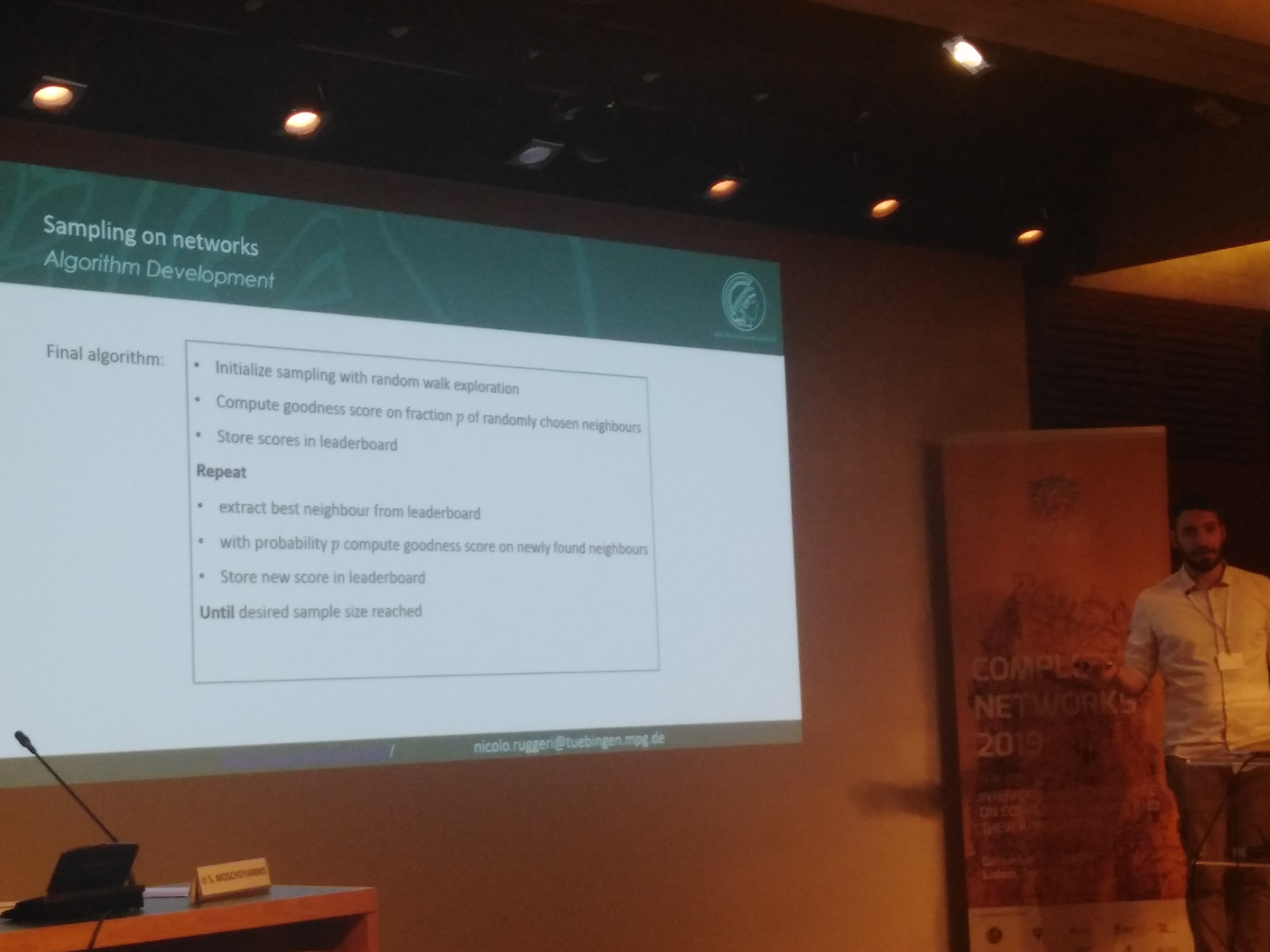
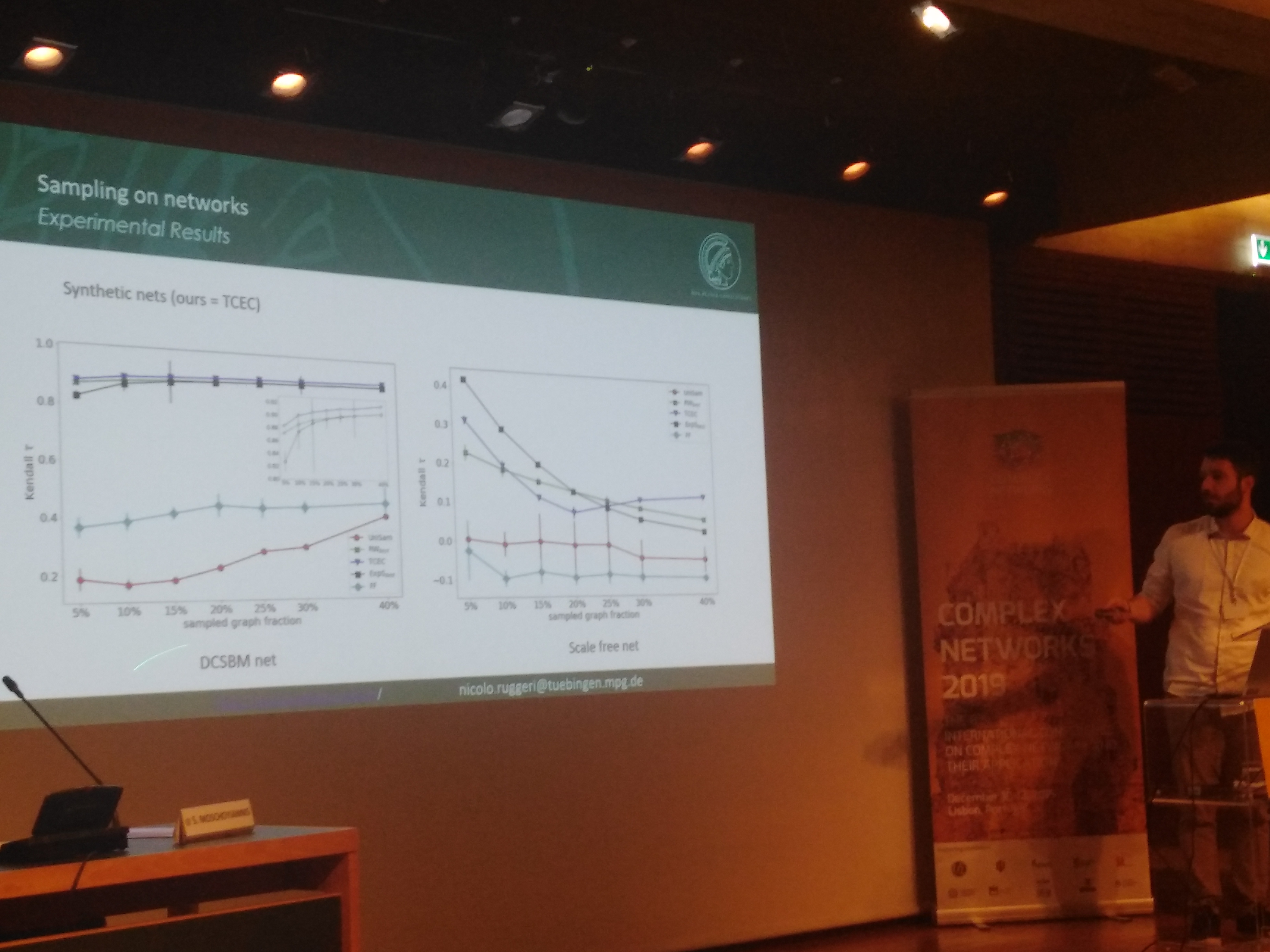
Enumerating isolated cliques in temporal networks
Hendrik Molter gave an interesting talk in formulating the retreival of isolated cliques in a temporal network. The main idea was to check all possible combinations of maximum-average cliques in different time patterns (usually-alltime). Moreover, the changes of an average-usual cliques are bound between consecutive time states with a constant. Subsequently, they provide a fixed-parameter enumeration algorithm and derive computational times for all aforementioned cases.
Deep Reinforcement Learning for Task-driven Discovery of Incomplete Networks
Peter Morales presented a novel reinforcement learning algorithm for network discovery.
The aim is to uncover hiden parts of the network by choosing nodes from a border set based on a policy learnt offline for an actor-critic model.
The training set for the policy is formed by simulated possible trajectiories, every trajectory having a certain background network, and every step corresponding to possible expansions of that network.
The policy is learnt by a neural network, and since the number of candidate nodes are too big in each step, they are ranked based on personalized pagerank first.
The results indicate that the method is superior to other methods that rely on online examples.
Moreover, the methods trained on simulated networks such as stochastic block models, generalizes very well to large scale real world networks such as Facebook.
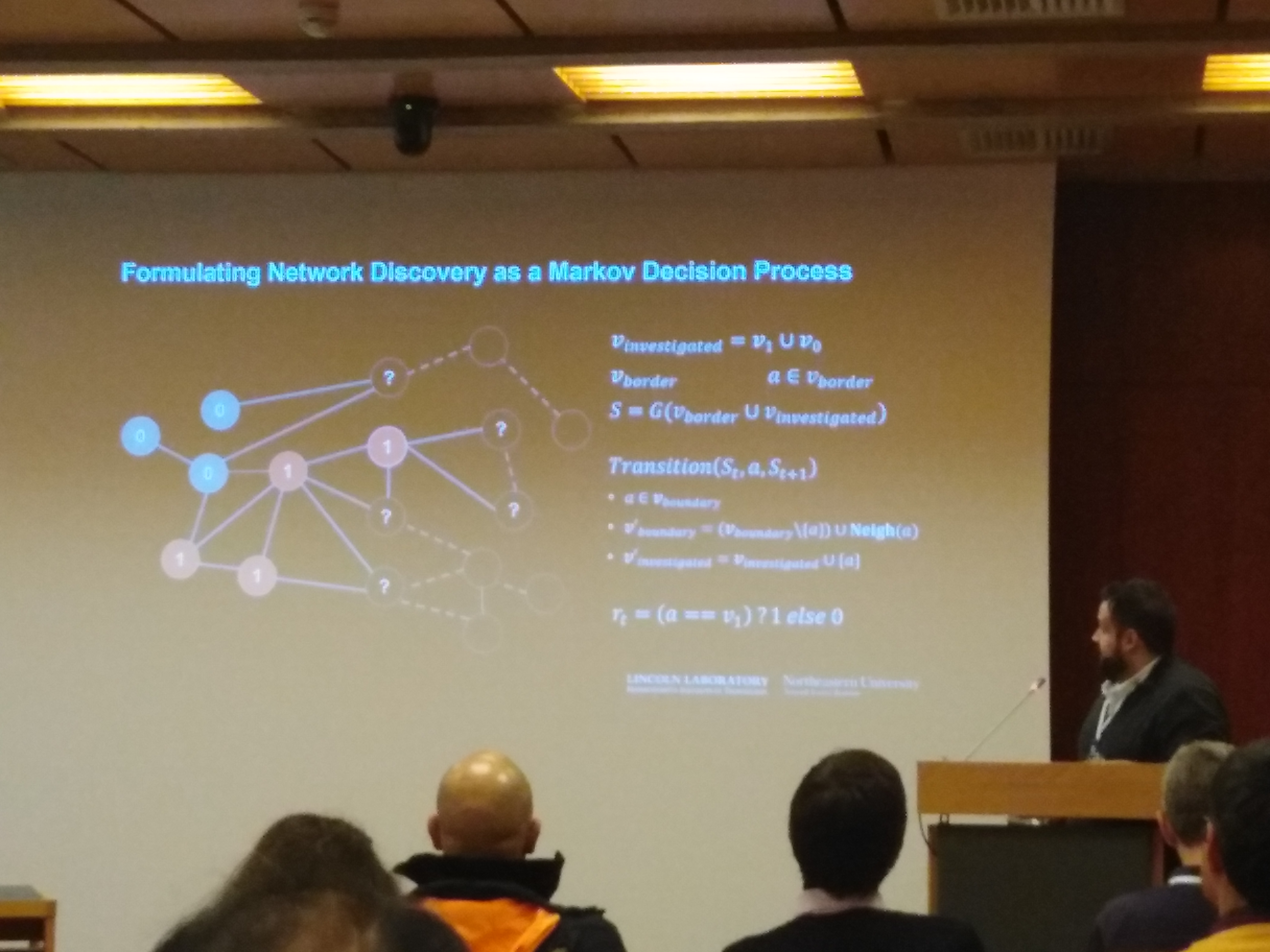
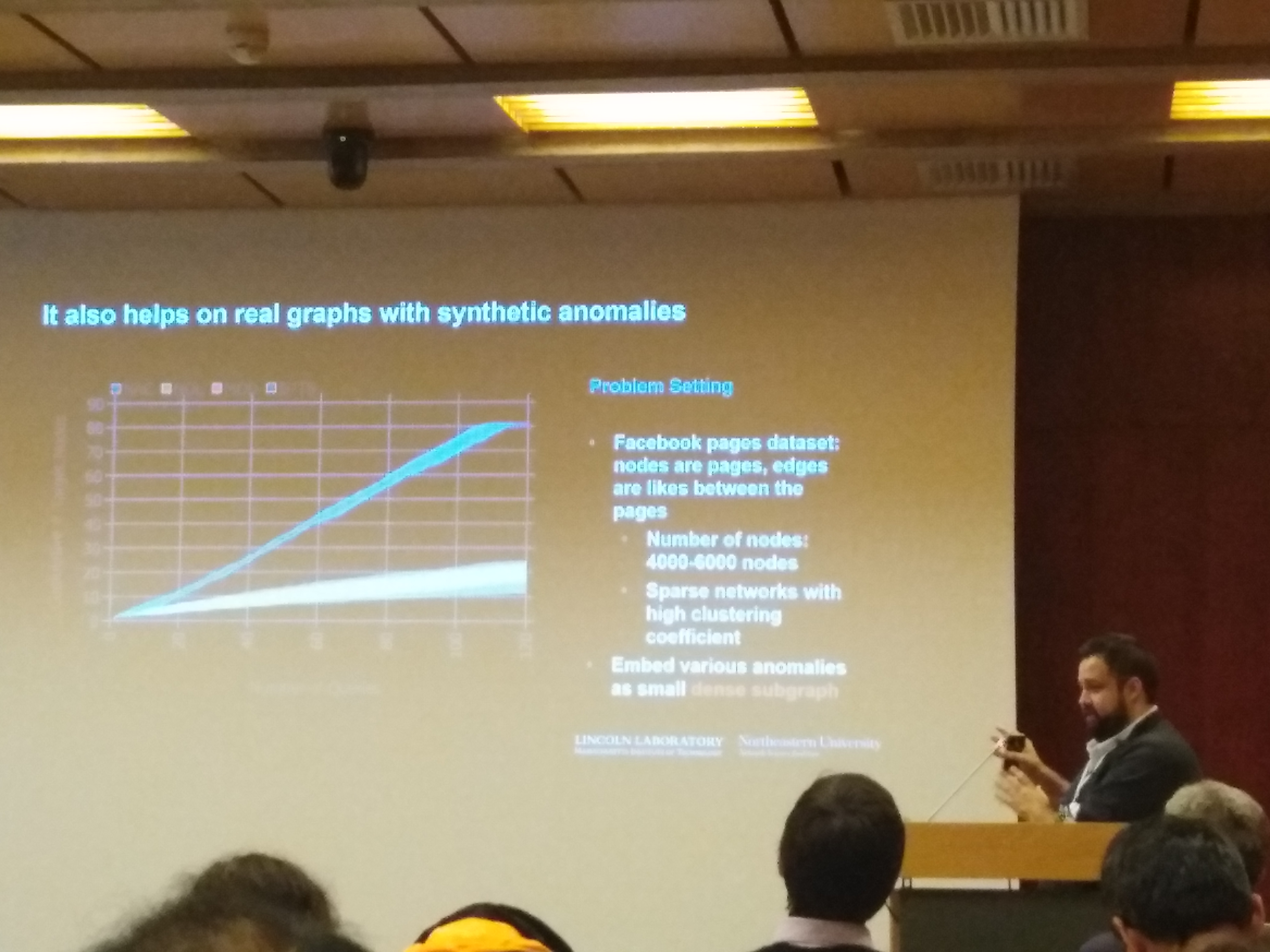
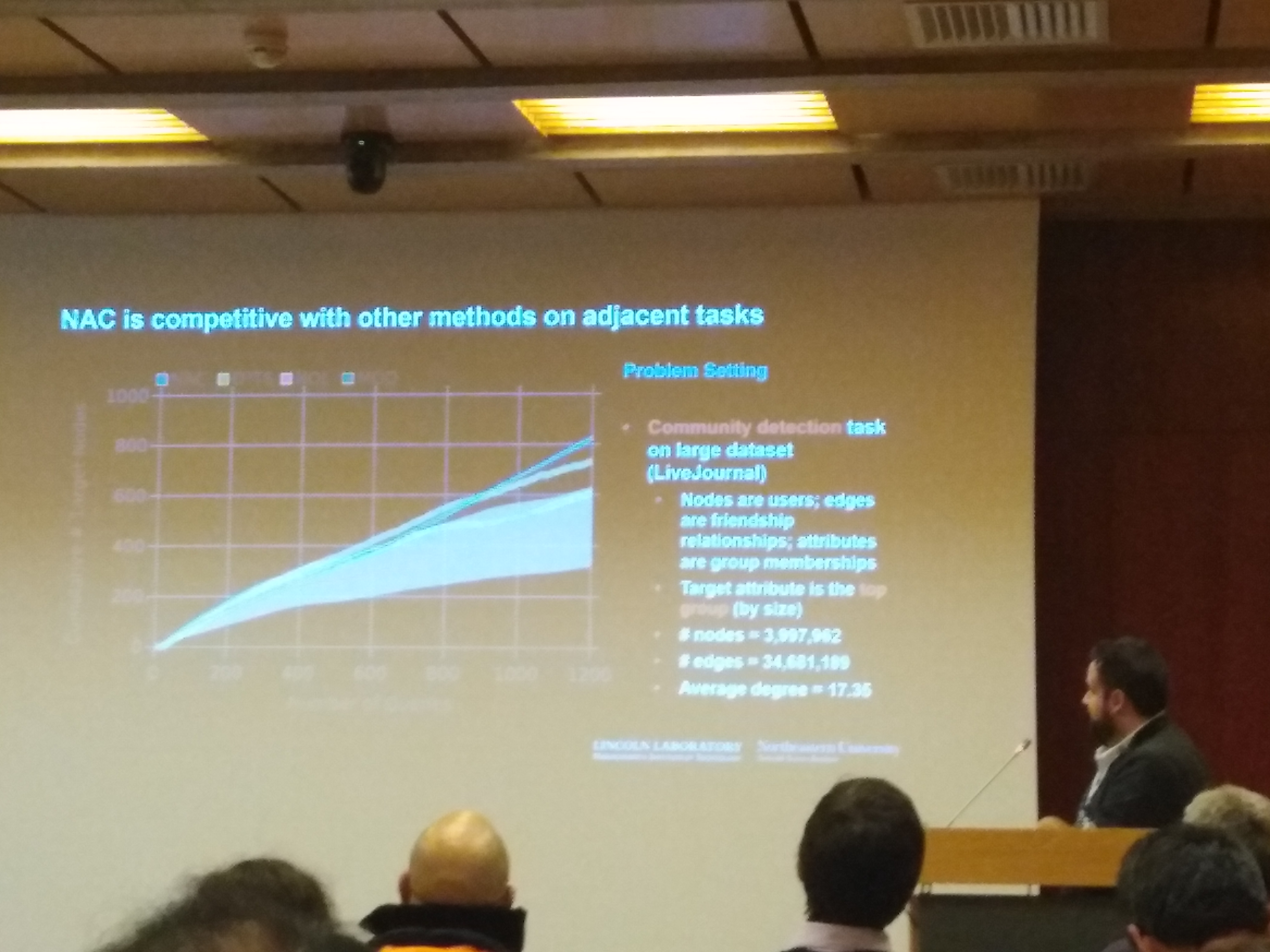
Quantifying predictability in Football through network analysis; A historical approach
An insightfull talk on predicting football (and why it’s so hard), was given by Prof. Taha Yasseri. Some of the main takes were that small country leagues are harder to predict then big ones, and home advantage is very crucial to a team’s overall performance.
Roles in social interactions: graphlets in temporal networks applied to learning analytics roles in social interactions: graphlets in temporal networks applied to learning analytics
Raphaël Charbey showcased a temporal analysis of social networks of students throughout an academic semester. The networks were formed by exercise validation between students, and it was broken down to snapshots based on sufficient time spaces of inactivity, in this case the threshold was 15 days. In each snapshot network graphlets of size 3 were derived, and node embeddings were derived based on the number of graphlets the node belongs to in each time step. Then the nodes were clustered based on those embeddings and their roles were defined, with some very cool visualizations.
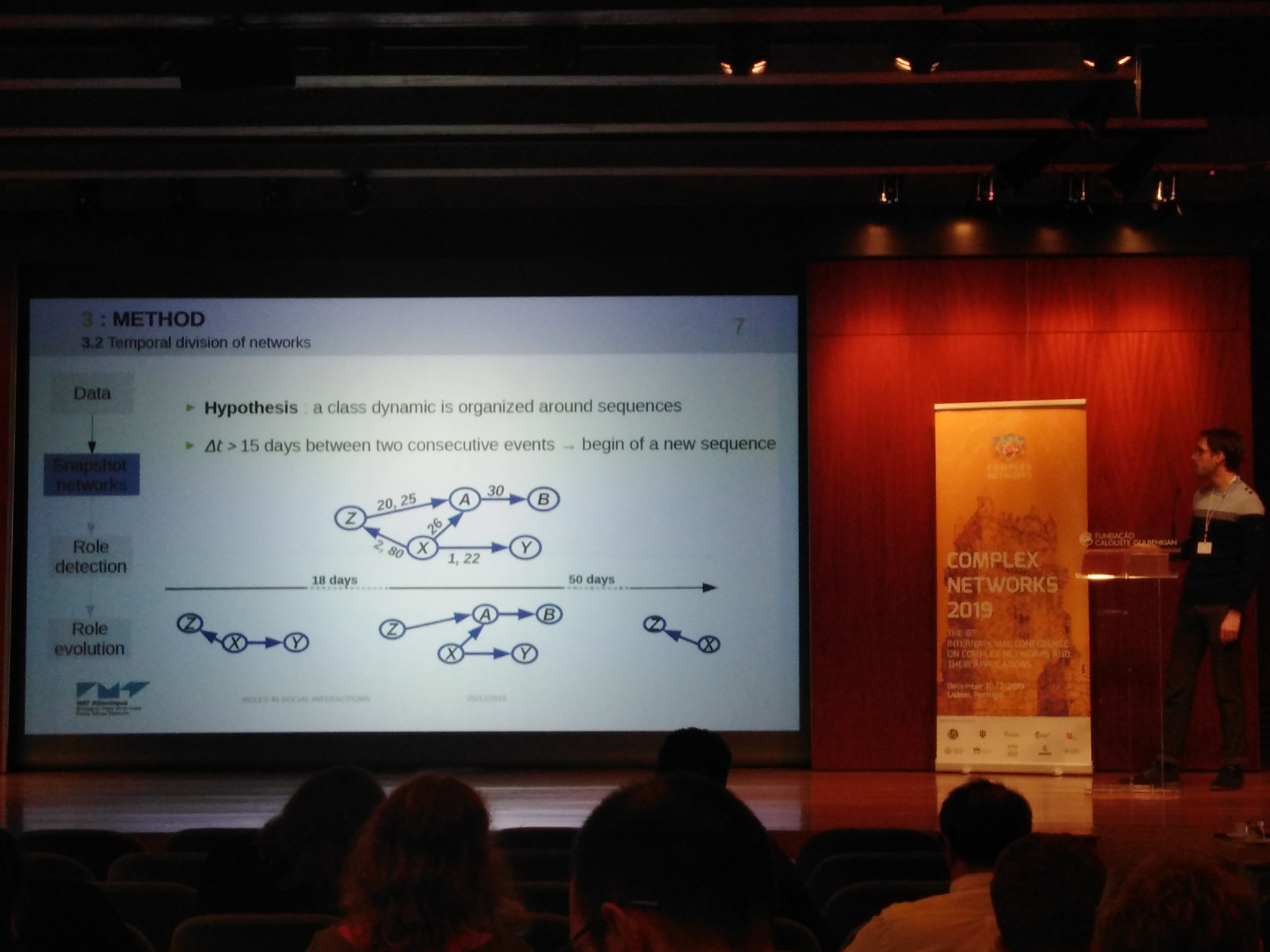
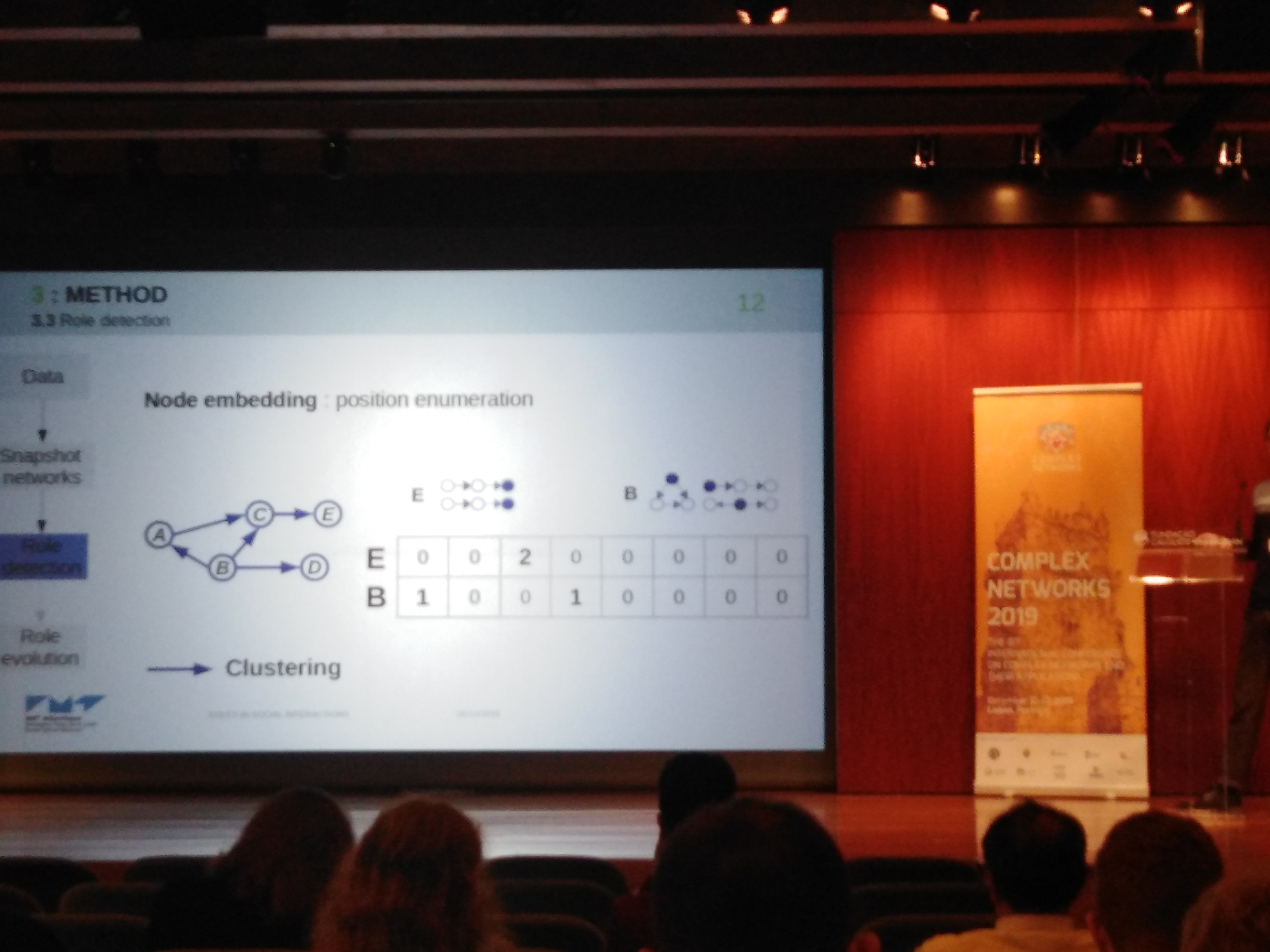
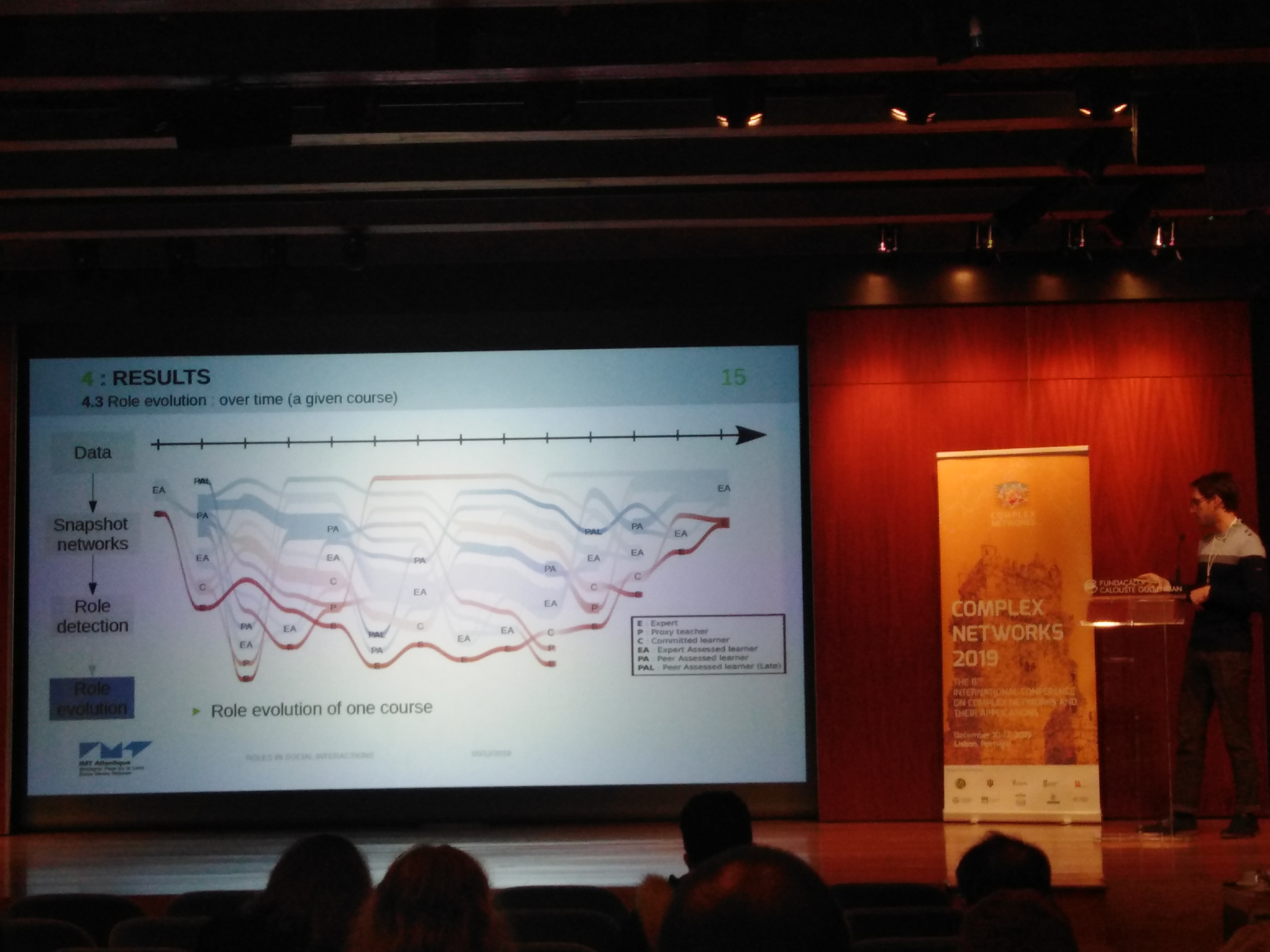
Transporters: Spring-systems in disguise. A physics model for analysing transporter networks
An impressive lighting talk by Jonathan Bourne about an embedding of networks based on spring-systems. This representation showcased high discriminative accuracy in simulated networks and insightfull plots in a real-world application.
A Simple Approach to Attributed Graph Embedding
Alberto Montresor presented a graph autoencoder, SAGE2VEC, where the input consists of network and attributal information. The aim of is to recunstruct a node’s neighborhood and its attributes, with a sparsity constraint. The results in four well-known datasets for node classification and link prediction are promising.
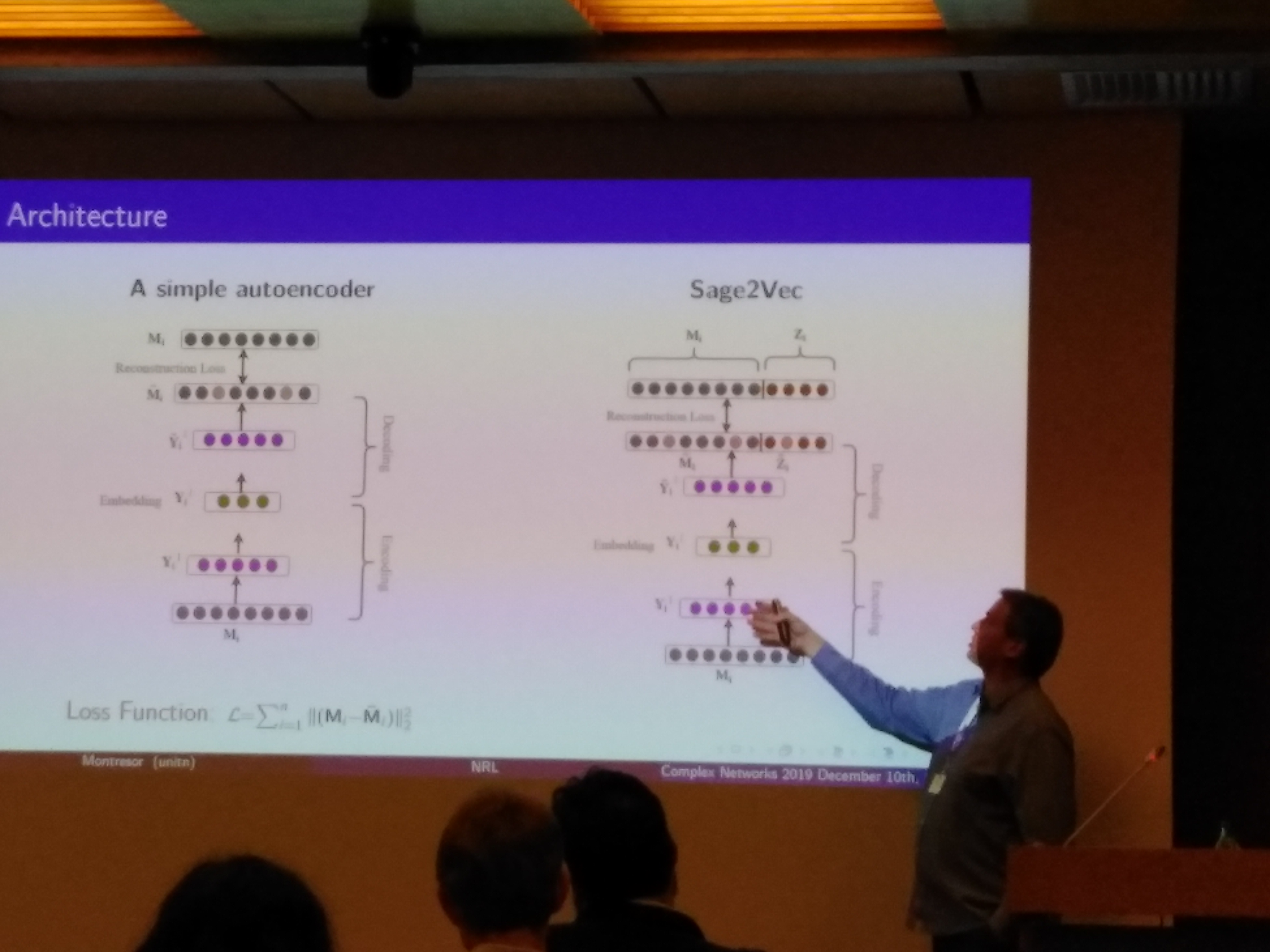
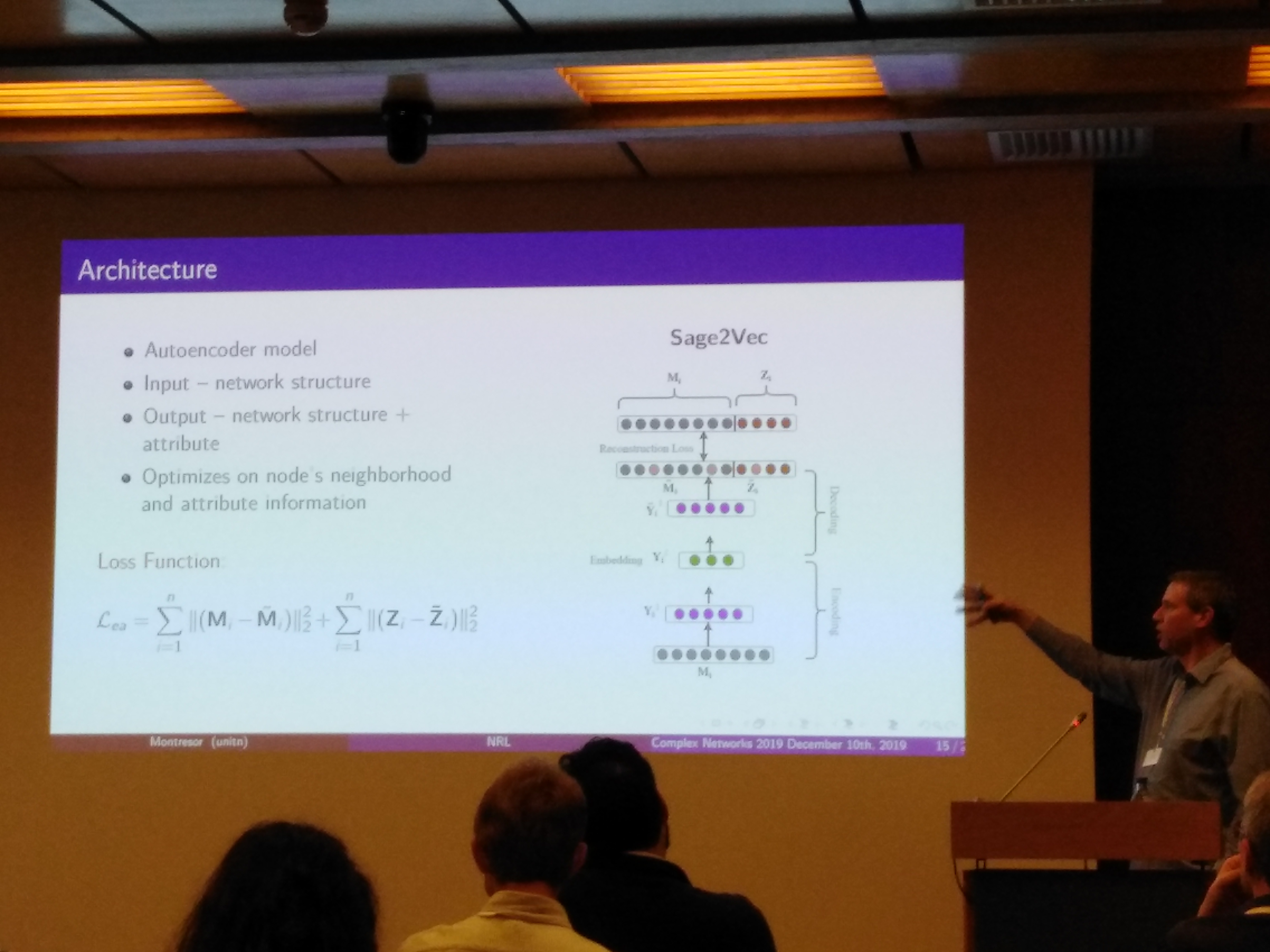
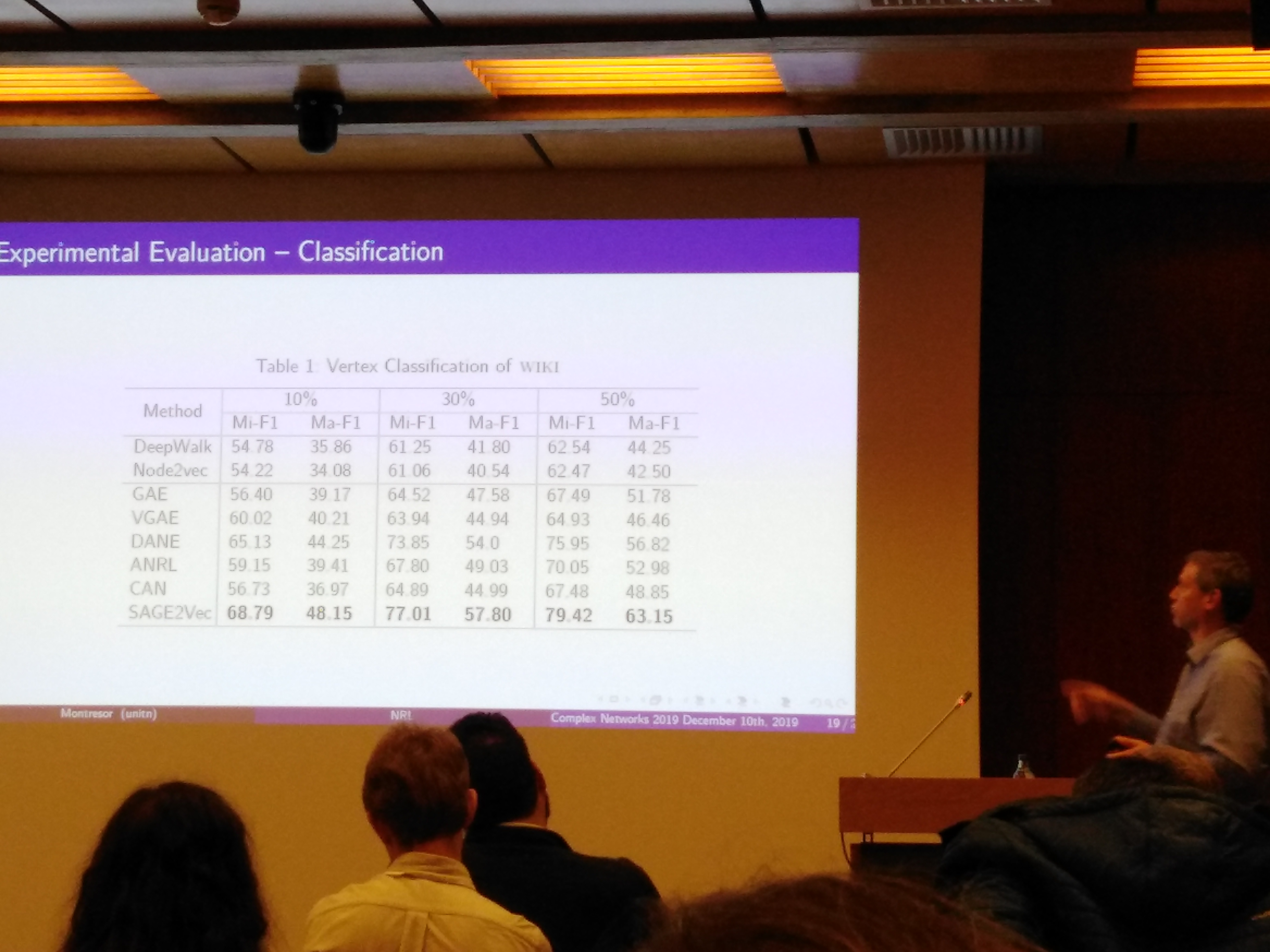
Community-Aware Content Diffusion: Embeddednes and Permeability
Giulio Rossetti, from the team of the creators of ndlib presented a model of diffusion that takes into account spreading inside and between communities. More specifically, the diffusion model has higher probability of passing the information between nodes inside the community rather then between communities. The difference in the rate of communities reached at the end of the simulation is significant.
Conclusion:
Overall I believe the conference achieves an increasing balance between computer science and physics approaches, as this year many submissions addressed problems using machine learning or big data, apart from several simulation or exploration based studies which is the central theme of network science. Lisbons is quite lively and easy to explore on foot. Bonus: a sand sculpture of a crocodile on the beach of Lisbon.
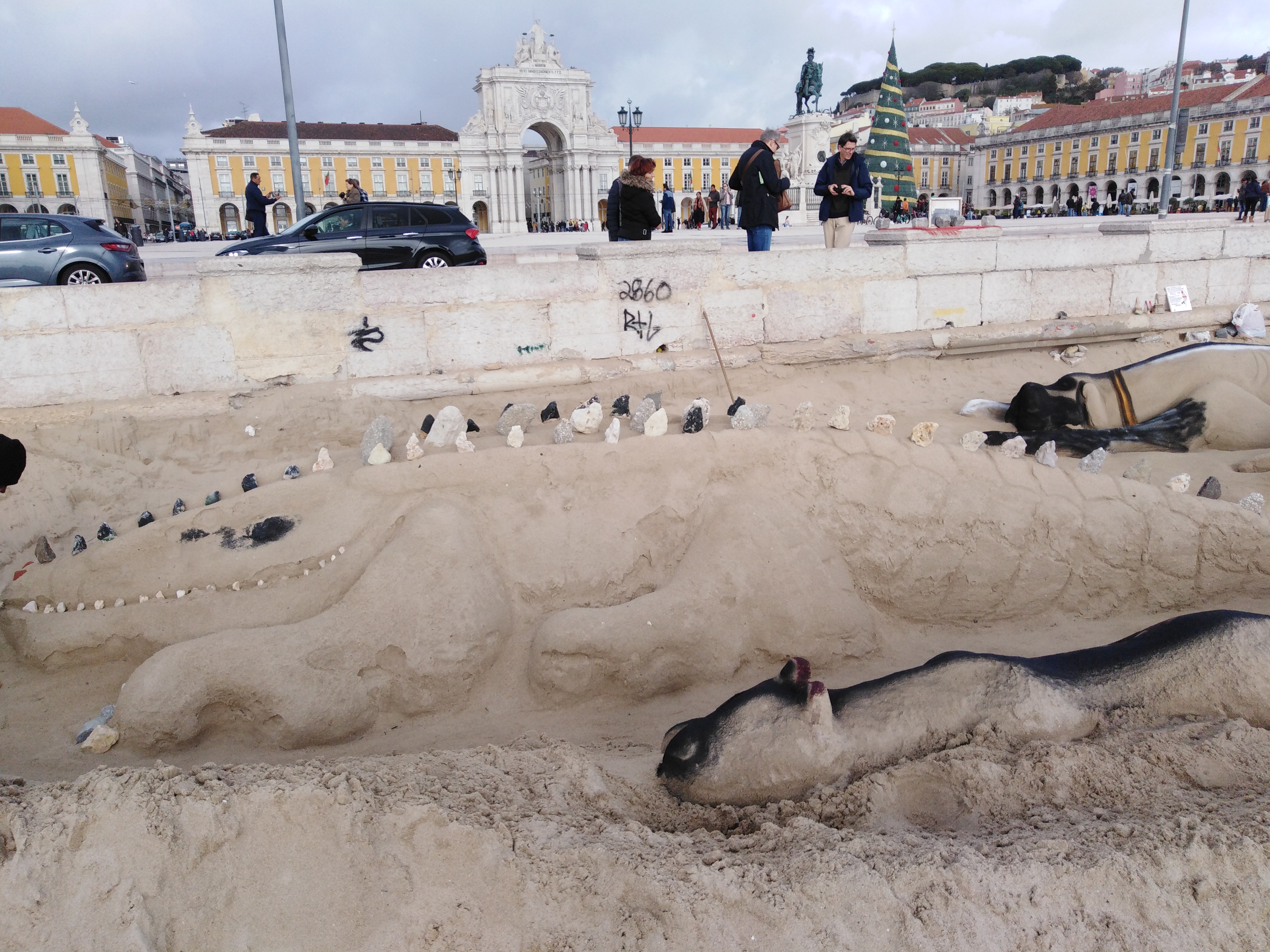